Which Industries Can Benefit from AI Development?
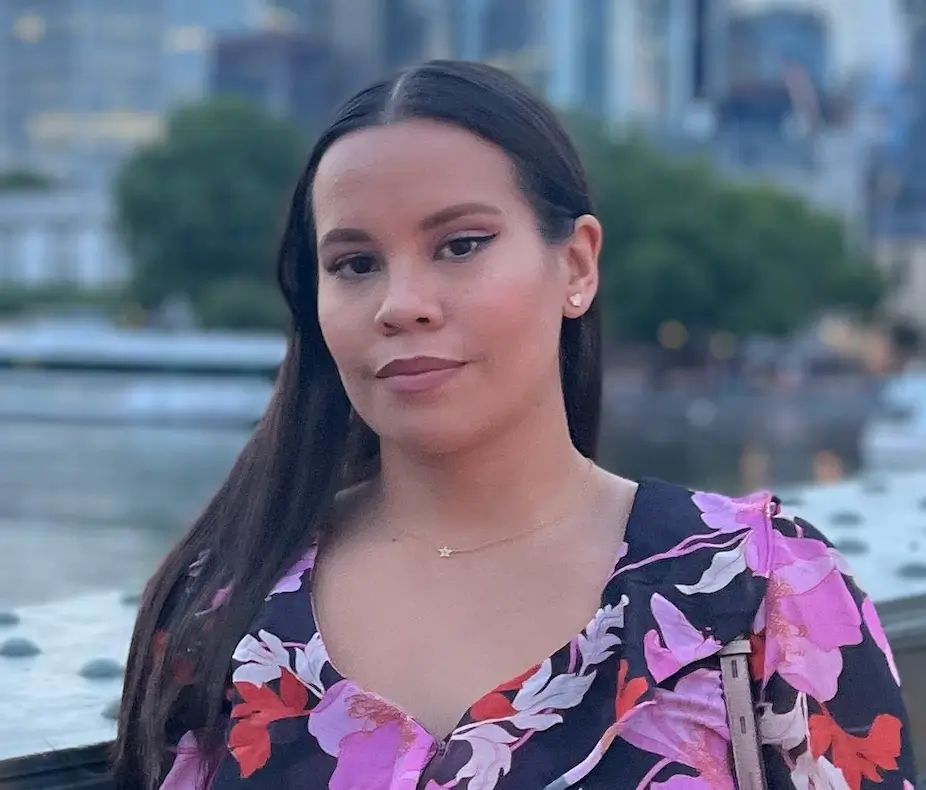

.avif)
Subscribe to our Newsletter
AI is no longer a futuristic concept, it’s already transforming how companies operate, compete, and deliver value. From automated workflows to predictive analytics and content generation, AI development now plays a practical role in nearly every major industry.
But while the potential is wide, not every use case is worth pursuing. Some sectors are seeing faster results, clearer returns, and better adoption than others.
In this blog, we’ll explore which industries are gaining the most from AI systems, how they’re applying tools like machine learning, natural language processing, and generative AI, and how to evaluate if your business is ready to implement AI in a way that actually works.
What Does AI Development Involve?
AI development is the process of designing, training, and deploying software systems that simulate aspects of human intelligence. These systems are built to solve specific problems, interpret natural language, make predictions, or automate tasks that would otherwise require manual effort.
AI can be organized based on its capabilities as weak AI and strong AI. It combines elements of computer science, data analytics, and machine learning.
Developers start by defining the problem, identifying the type of AI solution needed, such as a recommendation engine, a virtual assistant, or an image classification model, and collecting the necessary training data.
Once the problem and data are defined, developers train AI models using algorithms designed for supervised, unsupervised, or reinforcement learning.
Supervised learning involves the use of labeled data sets to train algorithms to classify data or predict outcomes accurately. This training process often involves deep neural networks, which use multiple layers to process unstructured data such as text, images, or audio.
These deep learning techniques are modeled in part on how the human brain interprets information, allowing systems to recognize patterns and improve accuracy over time.
Depending on the use case, the development process may involve:
- Natural language processing (NLP) for analyzing and generating human-like responses
- Computer vision for detecting and classifying visual input
- Predictive analytics for forecasting based on historical data
- Generative AI for producing content, simulations, or new data outputs
Successful AI development also requires clean, quality data, well-defined goals, and continuous evaluation of system performance.
Ethical considerations, like fairness and transparency, are now a standard part of responsible development workflows. AI ethics focuses on optimizing the beneficial impact of AI while reducing risks and adverse outcomes.
Differences Between Traditional Software and AI Solutions
While traditional software relies on fixed logic and predefined instructions, AI solutions are built to learn, adapt, and improve over time. The two approaches serve different purposes, and understanding the difference helps companies choose the right tool for the specific problem they are solving.
Traditional software development uses explicit rules to perform repeatable tasks.
A developer writes step-by-step code that the program follows every time it runs. These systems are ideal when workflows are stable, inputs are predictable, and decisions do not require ambiguity or real-time adjustments. Banking systems, form validation, and static dashboards are examples.
In contrast, AI development focuses on building systems that perform tasks typically requiring human intelligence, such as identifying objects in images, interpreting natural language, or adapting to changing inputs.
Instead of being told exactly what to do, AI models are trained on diverse datasets and improve performance by recognizing patterns, making predictions, and adjusting their behavior based on outcomes.
Key distinctions include:
- Logic vs. learning: Traditional apps follow rules. AI systems learn from training data using machine learning algorithms.
- Static behavior vs. adaptability: Once deployed, traditional systems don’t evolve. AI tools adjust based on new input, user behavior, or feedback.
- Predictability vs. pattern recognition: Traditional software delivers consistent output. AI systems use deep learning techniques to respond to ambiguity and unstructured data.
- Manual updates vs. autonomous refinement: Traditional apps need manual updates to change behavior. AI solutions can refine predictions using reinforcement or unsupervised learning strategies.
Modern artificial intelligence systems, such as those used in image recognition, virtual assistants, and predictive analytics, use techniques like neural networks and deep learning to simulate decision-making based on how the human brain processes information.
Generative adversarial networks (GANs) involve two neural networks competing against each other to improve output accuracy, further enhancing AI capabilities.
While traditional software remains essential, AI introduces a layer of intelligence that enables advanced systems to assist with problem-solving, automate decisions, and operate at scale with minimal human intervention.
Industries Rapidly Adopting Artificial Intelligence
Healthcare: Deep Learning & Computer Vision AI
Healthcare is one of the most transformative fields for AI development, where deep learning and computer vision are improving diagnostics, facilitating operations, and expanding access to care.
Generative AI has potential applications in various industries, including healthcare and marketing, for content personalization. These AI systems are trained to assist medical professionals with tasks that require speed, accuracy, and the ability to interpret large volumes of data.
Hospitals and research institutions use deep neural networks to interpret medical scans, such as MRIs, CTs, and X-rays, with high precision. These models excel at image recognition, identifying patterns that may be overlooked in fast-paced or high-pressure environments.
Tools built with deep learning algorithms can detect early signs of disease, measure anomalies, and support clinical decision-making with consistent accuracy.
In addition to visual tasks, natural language processing is helping healthcare teams analyze physician notes, lab reports, and patient records.
Key applications include:
- Tumor detection using artificial neural networks
- Predictive models for treatment outcomes and readmission risks
- Language analysis tools that surface red flags in patient histories
- AI-driven triage assistants that suggest next steps based on symptoms
These tools do not replace clinicians, but instead act as AI agents that augment human expertise.
With the right training data and oversight, they reduce the margin for error and speed up processes that were previously manual and time-consuming.
As privacy, safety, and accountability remain top concerns, healthcare AI also benefits from active research into responsible AI and the design of ethical models. These efforts help ensure that AI algorithms support patient safety while respecting confidentiality and regulatory standards.
Finance: Machine Learning & Natural Language Processing (NLP)
In finance, AI development is adapting how institutions handle risk, detect fraud, and serve customers at scale.
Two major technologies, machine learning and natural language processing (NLP), are at the center of this change, helping firms to process large volumes of data while minimizing manual review and human error.
Machine learning models trained on historical data can identify spending patterns, detect anomalies in transactions, and forecast credit risk with more precision than traditional scoring systems. These models continually improve, allowing institutions to respond effectively to growing fraud tactics and shifting economic conditions without requiring constant manual adjustments.
Meanwhile, NLP tools are helping banks, lenders, and fintech platforms make sense of unstructured communication.
Customer support transcripts, loan applications, and regulatory documents are processed using natural language algorithms that understand tone, intent, and sentiment. These systems can summarize complex text, identify key risks, and even flag compliance issues in real-time.
Use cases in finance include:
- Real-time fraud detection using machine learning algorithms
- Automated underwriting and credit scoring
- AI chat systems for client support, powered by natural language models
- Regulatory compliance monitoring through document analysis
Behind the scenes, these tools rely on robust training data, clean data collection processes, and oversight from data scientists and compliance teams.
Retail: Recommendation Systems & Generative AI
In retail, AI development is helping companies create highly personalized shopping experiences through intelligent systems that adjust to user behavior in real-time. AI can automate workflows and processes, such as monitoring and analyzing network traffic in cybersecurity.
Two technologies driving this transformation are recommendation engines and generative AI.
Recommendation systems rely on machine learning models to analyze browsing habits, past purchases, and product preferences. By recognizing patterns in customer data, these tools suggest items likely to convert, whether it's showing matching products, restocking reminders, or cross-category bundles.
Unlike traditional filters, these models continuously improve their suggestions using live feedback and new data collection points.
Meanwhile, generative AI capabilities are helping retailers create content at scale. Product descriptions, marketing copy, and customer prompts can be written automatically using natural language processing and deep learning techniques.
Uses of AI in retail include:
- Dynamic pricing based on demand and inventory trends
- Personalized email and website messaging
- Search functions that understand phrasing in human language
- Visual product search using image recognition tools
These systems are supported by deep neural networks trained on diverse data, enabling retailers to deliver suggestions and experiences tailored to individual shoppers, rather than just broad categories.
Some platforms also use reinforcement learning to adjust strategies based on which items users engage with or ignore.
This reduces decision fatigue, increases time on site, and improves conversions. For teams managing thousands of products and users, it also saves time previously spent on manual tagging or campaign setup.
Manufacturing: Predictive Maintenance AI & Robotics
The manufacturing industry relies on efficiency, consistency, and uptime. AI development is giving factories the tools to prevent costly delays, improve equipment performance, and increase output through systems that anticipate problems and automate repetitive tasks.
Predictive maintenance uses machine learning models to monitor machinery and detect early signs of wear.
These systems analyze data from sensors embedded in production lines to forecast when a machine is likely to fail. Instead of reacting to breakdowns, teams can schedule repairs in advance to prevent production interruptions.
These systems are trained on historical data, then refined through continuous data collection. By recognizing subtle changes in vibration, temperature, or output speed, they provide alerts that allow teams to act precisely when needed.
Alongside monitoring, robotic process automation and AI-powered machines are improving how materials are handled and assembled.
Some factories deploy robots guided by deep learning algorithms, which are capable of identifying defects, adapting to object positions, and assisting workers with tasks that require speed and precision.
Some examples include:
- Quality control with vision-guided inspection tools
- Assembly line assistance using robotic arms
- Predicting inventory demand based on live production rates
- Load balancing in energy or material usage
The combination of artificial neural networks and predictive analytics allows for fast decisions with minimal human intervention, supporting safer environments and better use of skilled labor.
Transportation & Logistics: Reinforcement Learning & Computer Vision
In transportation and logistics, AI development is helping companies move goods more efficiently, plan routes with greater precision, and reduce dependency on manual tracking. AI is utilized in smart city applications to optimize resource management and improve urban services.
Two technologies are proving especially useful: reinforcement learning and computer vision.
Reinforcement learning allows AI systems to improve through trial and reward. These models simulate thousands of delivery scenarios, learning which routes, sequences, or methods produce the best outcomes in terms of time, fuel use, and delivery accuracy.
Over time, this process helps optimize decisions around fleet distribution, traffic conditions, and last-mile coordination, without requiring fixed rules.
For visual tasks, computer vision is applied to monitor vehicles, scan barcodes, verify loads, and assess road conditions in real time. Using deep learning models, these systems process video or image inputs to identify obstacles, detect anomalies, and confirm safe handoffs.
In warehouses, vision-based robotics assists with sorting, packaging, and inventory verification.
Common use cases include:
- Route optimization for delivery networks
- Cargo monitoring using real-time camera feeds
- Lane tracking and hazard detection in autonomous vehicles
- Robotic assistance in loading zones and fulfillment centers
These tools are most effective when trained on high-quality data and integrated with tracking systems. They also support resource management by reducing time lost to idle vehicles, delayed shipments, or human error in task execution.
Legal & Compliance: NLP & Document Intelligence AI
Legal and compliance teams are turning to AI development to manage increasingly large volumes of documentation, detect risks, and ensure regulatory accuracy without expanding headcount. AI tools have been used to enhance customer service through chatbots and virtual assistants that operate 24/7.
Two technologies leading this transformation are natural language processing (NLP) and document intelligence systems.
NLP-based models help companies extract meaning from legal language, summarizing contracts, flagging clauses, and surfacing inconsistencies. These systems interpret complex phrasing in human language, reducing time spent reviewing documents manually.
Document intelligence tools, powered by deep learning models, use structured and unstructured data to classify, tag, and organize files across formats. When integrated with case management software or cloud storage, they improve search accuracy, speed up audits, and help track legal obligations in real time.
Practical applications include:
- Contract analysis and clause comparison
- Regulatory document review across jurisdictions
- Discovery support during litigation
- Real-time policy monitoring in regulated industries
These solutions rely on well-trained machine learning algorithms, supported by high-quality data and updated to reflect the latest legal standards.
Education: Adaptive Learning AI & Generative AI
In education, AI development is unlocking new ways to personalize learning and improve how students engage with material. Through adaptive learning systems and generative AI, schools and edtech platforms are moving beyond static lesson plans and one-size-fits-all content.
Generative AI can create original content such as text, images, and videos in response to user prompts.
Adaptive learning tools adjust in real time based on how each student interacts with questions, videos, and activities.
Using machine learning models, these platforms track performance, identify learning gaps, and deliver material tailored to that student’s progress. The system learns from responses and guides the learner at the right pace, reducing frustration and reinforcing understanding.
On the content side, generative AI is helping educators create quizzes, practice questions, and summaries automatically.
Built on deep learning models and language-based AI algorithms, these tools understand curriculum structure and produce content aligned with specific goals. Some platforms use these models to simulate interactive tutoring experiences, offering explanations in different formats depending on the learner’s preferences.
Use cases include:
- Personalized study plans based on performance data
- AI-generated writing prompts or feedback suggestions
- Adaptive testing tools that adjust difficulty dynamically
- Virtual tutors who answer follow-up questions in plain language
Behind the scenes, these systems are powered by neural networks, training data, and ongoing data analytics. They do not replace instructors, but extend what educators can do, especially in large classrooms or remote learning environments.
Main Benefits of AI Systems
Faster Decision-Making with Real-Time Data
Modern AI systems process and respond to incoming data far faster than traditional workflows.
In environments where speed determines outcomes, finance, logistics, manufacturing, this advantage is hard to ignore. Through the use of machine learning algorithms and neural networks, these tools track, interpret, and react to high-volume inputs without pause.
Trained on quality data and connected to live feeds, AI models assist with forecasting, route planning, supply monitoring, and fraud detection.
In each case, the model’s ability to analyze data instantly helps decision-makers act without delay.
Examples include:
- Market trend reactions in financial platforms
- Real-time risk alerts in cybersecurity
- Adjustments in supply chains based on delivery status
Reduced Human Error in High-Stakes Environments
Mistakes in fields like aviation, healthcare, or infrastructure can lead to serious consequences. AI development is helping reduce these risks by introducing tools that follow consistent logic, maintain full attention on data, and avoid fatigue.
AI algorithms can embed ethical considerations and value choices into their programming, impacting decision-making. AI tools, when trained properly, minimize oversight by recognizing patterns and flagging inconsistencies early.
In healthcare, deep learning models identify anomalies in diagnostic scans. In transportation, automated systems support drivers with warnings, lane tracking, and braking assistance.
These systems don’t rely on memory or guesswork. Once built, they evaluate situations based on defined parameters, historical outcomes, and thousands of previous data points.
AI doesn’t eliminate all mistakes, but when applied correctly, it reduces the chance of oversight and improves safety in places where accuracy matters most.
Personalized Experiences at Scale
Delivering individual-level experiences used to require extensive manual effort.
Now, AI systems powered by machine learning algorithms can adjust responses, content, and recommendations for each user, without increasing the workload.
By analyzing behavior, past interactions, and unstructured data sets, these models create tailored outputs across industries. In e-commerce, they suggest products that match previous purchases.
In education, lessons are adjusted to the learner’s pace. In the media, they curate content based on user preferences and the time of day.
The engine behind this flexibility includes:
- Deep learning models capable of interpreting subtle usage patterns
- Text and voice understanding through natural language processing
- Real-time adjustments based on feedback or changes in context
Increased Efficiency in Routine Operations
Many tasks within business operations repeat daily, from responding to support tickets to generating reports. AI development helps reduce the burden of these functions by automating processes that don’t require human judgment.
Trained AI models process these tasks using clear logic and defined rules, making them ideal for structured decisions and data analysis.
This includes categorizing emails, handling basic requests, flagging anomalies, and sorting documents.
Tools used include:
- Workflow automation through API-driven bots
- Virtual assistants that respond to common questions
- Systems that fill forms or transfer data between platforms
How to Evaluate AI Fit for Your Industry
Does Your Business Generate High Volumes of Data?
Industries that collect large amounts of information, structured or unstructured, stand to gain the most from AI systems. Whether you're logging transactions, customer interactions, machine output, or logistics data, volume is a clear indicator of potential.
AI development becomes valuable when there is sufficient input to train models effectively. Machine learning algorithms rely on consistent access to high-quality data to identify patterns, test predictions, and produce reliable outcomes.
Examples include:
- Retail platforms tracking thousands of daily purchases
- Manufacturing teams recording sensor data from equipment
- Financial services processing transaction histories in real time
If your operation involves continuous data collection, there is likely an opportunity to use AI models for optimization, predicting, or anomaly detection. The more consistent the flow, the stronger the potential for automation and insight generation.
Are There Tasks That Require Repetition or Pattern Recognition?
Businesses often rely on employees to perform the same action hundreds of times each day, such as responding to similar messages, sorting entries, reviewing logs, or updating records.
These types of duties are well-suited for artificial intelligence.
AI systems excel at tasks that follow rules, repeat frequently, or involve identifying patterns in large datasets of information. Neural networks and deep learning models are especially effective at processing routine visual checks, language parsing, or form classification without fatigue or inconsistency.
Look for areas like:
- Processing invoices or receipts
- Analyzing support tickets for tone or urgency
- Reviewing sensor data for deviations in expected output
When tasks meet both criteria, high volume and recognizable patterns, it’s a clear indication that AI can reduce effort, speed up response times, and improve accuracy with minimal human intervention.
What Are the Risks of Manual Processing?
When tasks are performed by hand, especially in high-volume or time-sensitive environments, the potential for mistakes increases. Manual work often lacks consistency, and errors can lead to delays, compliance failures, or added costs.
AI systems offer a way to reduce those risks through logic-based, repeatable processes that maintain accuracy at scale.
Some of the most common pain points caused by manual workflows include:
- Inconsistent data entry across departments
- Delayed responses due to task bottlenecks
- Missed insights buried in overlooked reports
In contrast, machine learning models and AI algorithms perform the same task with the same standard each time. They don’t lose focus, skip steps, or mislabel information.
Do You Have Clear Metrics to Track AI ROI?
Successful AI development depends on more than the model itself, it also relies on knowing how to measure impact. Companies need clear, business-aligned benchmarks that reflect what the system is meant to improve.
Before starting any project, define what outcomes matter most.
That might be faster response times, fewer support requests, higher forecast accuracy, or increased output with the same team size. Once these targets are set, AI systems can be monitored through a combination of KPIs and real-time performance logs.
Strong signals of ROI readiness include:
- Clear baselines for current performance
- Available quality data for historical comparison
- A way to monitor AI output using analytics or logs
When goals are measurable, machine learning tools can be assessed properly and refined over time using insights from data analysis. Without metrics, it is difficult to determine whether automation is actually beneficial or merely adding complexity.
NerdHeadz Builds Industry-Specific AI Tools
At NerdHeadz, we help businesses bring AI development from idea to execution, focusing on real-world use cases instead of vague promises.
Our team works directly with companies across various industries, including logistics, education, retail, and services, to build tools that solve specific challenges, not just showcase the technology.
Each project begins by understanding your workflow.
We examine what is being done manually, where time is wasted, and which decisions rely on repetitive judgment. From there, we build AI systems tailored to those patterns, whether it means automating triage, generating content, analyzing incoming data, or delivering more responsive user experiences.
Some of the custom software we’ve built include:
- AI bots that qualify leads for real estate platforms
- Scheduling assistants for educators with built-in follow-up prompts
- Logistics dashboards with predictive insights and smart routing logic
- AI-powered document processors for compliance-heavy teams
- Onboarding flows with virtual assistants that adapt to user input
If your team needs industry-specific AI tools that go beyond experimentation, we’ll help you build something useful, measurable, and built for your next stage of growth, and to attract early adopters.
Conclusion
The industries seeing the most from AI development aren’t just adopting it because it’s trending, they’re using it to solve specific problems faster, more accurately, and with less manual effort. Whether it’s predicting machine failures, reducing customer wait times, or personalizing digital experiences, AI systems are helping teams improve outcomes and free up time for work that matters.
But success depends on building solutions that match your business, not a generic tool or one-size-fits-all model. That’s where we come in.
At NerdHeadz, we design industry-specific AI tools that deliver measurable impact from day one.
If you're ready to explore what AI can do for your team, book a call with us. We’ll help you find the right starting point, and build something that fits the way you already work.
Frequently asked questions
Which industry needs AI the most?
Industries with high volumes of data and repetitive tasks, such as healthcare, logistics, and finance, have the most urgent need for AI to improve speed, accuracy, and decision-making.
Which industries are investing the most in AI?
Finance, SaaS, manufacturing, and healthcare are leading sectors in AI investment, driven by use cases such as automation, predictive analytics, fraud detection, and operational efficiency.
Which industry is likely to benefit the most from generative AI?
Media, education, and marketing benefit significantly from generative AI due to the high demand for content. It’s also gaining traction in legal, customer service, and product design.
What stocks will benefit most from AI?
Major beneficiaries include companies in the semiconductor industry (such as NVIDIA), cloud infrastructure providers (like Microsoft or Amazon), and AI software platforms focused on automation and analytics.
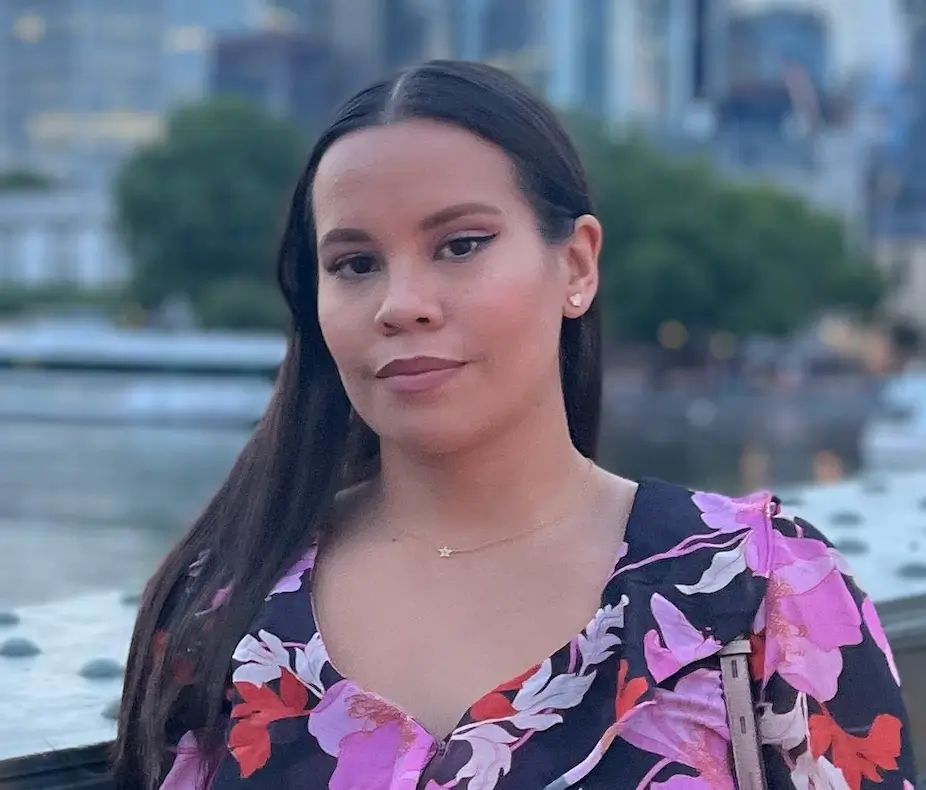
Luciani Zorrilla
Luciani Zorrilla is a content marketer with experience in sales development, outbound sales, SEO, design, email marketing, and UX. She stands out in driving sustainable growth for tech startups through impactful SEO strategies and leading results-oriented marketing teams.
Subscribe to our Newsletter
Are you ready to talk about your project?
Schedule a consultation with our team, and we’ll send a custom proposal.

AI is no longer a futuristic concept, it’s already transforming how companies operate, compete, and deliver value. From automated workflows to predictive analytics and content generation, AI development now plays a practical role in nearly every major industry.
But while the potential is wide, not every use case is worth pursuing. Some sectors are seeing faster results, clearer returns, and better adoption than others.
In this blog, we’ll explore which industries are gaining the most from AI systems, how they’re applying tools like machine learning, natural language processing, and generative AI, and how to evaluate if your business is ready to implement AI in a way that actually works.
What Does AI Development Involve?
AI development is the process of designing, training, and deploying software systems that simulate aspects of human intelligence. These systems are built to solve specific problems, interpret natural language, make predictions, or automate tasks that would otherwise require manual effort.
AI can be organized based on its capabilities as weak AI and strong AI. It combines elements of computer science, data analytics, and machine learning.
Developers start by defining the problem, identifying the type of AI solution needed, such as a recommendation engine, a virtual assistant, or an image classification model, and collecting the necessary training data.
Once the problem and data are defined, developers train AI models using algorithms designed for supervised, unsupervised, or reinforcement learning.
Supervised learning involves the use of labeled data sets to train algorithms to classify data or predict outcomes accurately. This training process often involves deep neural networks, which use multiple layers to process unstructured data such as text, images, or audio.
These deep learning techniques are modeled in part on how the human brain interprets information, allowing systems to recognize patterns and improve accuracy over time.
Depending on the use case, the development process may involve:
- Natural language processing (NLP) for analyzing and generating human-like responses
- Computer vision for detecting and classifying visual input
- Predictive analytics for forecasting based on historical data
- Generative AI for producing content, simulations, or new data outputs
Successful AI development also requires clean, quality data, well-defined goals, and continuous evaluation of system performance.
Ethical considerations, like fairness and transparency, are now a standard part of responsible development workflows. AI ethics focuses on optimizing the beneficial impact of AI while reducing risks and adverse outcomes.
Differences Between Traditional Software and AI Solutions
While traditional software relies on fixed logic and predefined instructions, AI solutions are built to learn, adapt, and improve over time. The two approaches serve different purposes, and understanding the difference helps companies choose the right tool for the specific problem they are solving.
Traditional software development uses explicit rules to perform repeatable tasks.
A developer writes step-by-step code that the program follows every time it runs. These systems are ideal when workflows are stable, inputs are predictable, and decisions do not require ambiguity or real-time adjustments. Banking systems, form validation, and static dashboards are examples.
In contrast, AI development focuses on building systems that perform tasks typically requiring human intelligence, such as identifying objects in images, interpreting natural language, or adapting to changing inputs.
Instead of being told exactly what to do, AI models are trained on diverse datasets and improve performance by recognizing patterns, making predictions, and adjusting their behavior based on outcomes.
Key distinctions include:
- Logic vs. learning: Traditional apps follow rules. AI systems learn from training data using machine learning algorithms.
- Static behavior vs. adaptability: Once deployed, traditional systems don’t evolve. AI tools adjust based on new input, user behavior, or feedback.
- Predictability vs. pattern recognition: Traditional software delivers consistent output. AI systems use deep learning techniques to respond to ambiguity and unstructured data.
- Manual updates vs. autonomous refinement: Traditional apps need manual updates to change behavior. AI solutions can refine predictions using reinforcement or unsupervised learning strategies.
Modern artificial intelligence systems, such as those used in image recognition, virtual assistants, and predictive analytics, use techniques like neural networks and deep learning to simulate decision-making based on how the human brain processes information.
Generative adversarial networks (GANs) involve two neural networks competing against each other to improve output accuracy, further enhancing AI capabilities.
While traditional software remains essential, AI introduces a layer of intelligence that enables advanced systems to assist with problem-solving, automate decisions, and operate at scale with minimal human intervention.
Industries Rapidly Adopting Artificial Intelligence
Healthcare: Deep Learning & Computer Vision AI
Healthcare is one of the most transformative fields for AI development, where deep learning and computer vision are improving diagnostics, facilitating operations, and expanding access to care.
Generative AI has potential applications in various industries, including healthcare and marketing, for content personalization. These AI systems are trained to assist medical professionals with tasks that require speed, accuracy, and the ability to interpret large volumes of data.
Hospitals and research institutions use deep neural networks to interpret medical scans, such as MRIs, CTs, and X-rays, with high precision. These models excel at image recognition, identifying patterns that may be overlooked in fast-paced or high-pressure environments.
Tools built with deep learning algorithms can detect early signs of disease, measure anomalies, and support clinical decision-making with consistent accuracy.
In addition to visual tasks, natural language processing is helping healthcare teams analyze physician notes, lab reports, and patient records.
Key applications include:
- Tumor detection using artificial neural networks
- Predictive models for treatment outcomes and readmission risks
- Language analysis tools that surface red flags in patient histories
- AI-driven triage assistants that suggest next steps based on symptoms
These tools do not replace clinicians, but instead act as AI agents that augment human expertise.
With the right training data and oversight, they reduce the margin for error and speed up processes that were previously manual and time-consuming.
As privacy, safety, and accountability remain top concerns, healthcare AI also benefits from active research into responsible AI and the design of ethical models. These efforts help ensure that AI algorithms support patient safety while respecting confidentiality and regulatory standards.
Finance: Machine Learning & Natural Language Processing (NLP)
In finance, AI development is adapting how institutions handle risk, detect fraud, and serve customers at scale.
Two major technologies, machine learning and natural language processing (NLP), are at the center of this change, helping firms to process large volumes of data while minimizing manual review and human error.
Machine learning models trained on historical data can identify spending patterns, detect anomalies in transactions, and forecast credit risk with more precision than traditional scoring systems. These models continually improve, allowing institutions to respond effectively to growing fraud tactics and shifting economic conditions without requiring constant manual adjustments.
Meanwhile, NLP tools are helping banks, lenders, and fintech platforms make sense of unstructured communication.
Customer support transcripts, loan applications, and regulatory documents are processed using natural language algorithms that understand tone, intent, and sentiment. These systems can summarize complex text, identify key risks, and even flag compliance issues in real-time.
Use cases in finance include:
- Real-time fraud detection using machine learning algorithms
- Automated underwriting and credit scoring
- AI chat systems for client support, powered by natural language models
- Regulatory compliance monitoring through document analysis
Behind the scenes, these tools rely on robust training data, clean data collection processes, and oversight from data scientists and compliance teams.
Retail: Recommendation Systems & Generative AI
In retail, AI development is helping companies create highly personalized shopping experiences through intelligent systems that adjust to user behavior in real-time. AI can automate workflows and processes, such as monitoring and analyzing network traffic in cybersecurity.
Two technologies driving this transformation are recommendation engines and generative AI.
Recommendation systems rely on machine learning models to analyze browsing habits, past purchases, and product preferences. By recognizing patterns in customer data, these tools suggest items likely to convert, whether it's showing matching products, restocking reminders, or cross-category bundles.
Unlike traditional filters, these models continuously improve their suggestions using live feedback and new data collection points.
Meanwhile, generative AI capabilities are helping retailers create content at scale. Product descriptions, marketing copy, and customer prompts can be written automatically using natural language processing and deep learning techniques.
Uses of AI in retail include:
- Dynamic pricing based on demand and inventory trends
- Personalized email and website messaging
- Search functions that understand phrasing in human language
- Visual product search using image recognition tools
These systems are supported by deep neural networks trained on diverse data, enabling retailers to deliver suggestions and experiences tailored to individual shoppers, rather than just broad categories.
Some platforms also use reinforcement learning to adjust strategies based on which items users engage with or ignore.
This reduces decision fatigue, increases time on site, and improves conversions. For teams managing thousands of products and users, it also saves time previously spent on manual tagging or campaign setup.
Manufacturing: Predictive Maintenance AI & Robotics
The manufacturing industry relies on efficiency, consistency, and uptime. AI development is giving factories the tools to prevent costly delays, improve equipment performance, and increase output through systems that anticipate problems and automate repetitive tasks.
Predictive maintenance uses machine learning models to monitor machinery and detect early signs of wear.
These systems analyze data from sensors embedded in production lines to forecast when a machine is likely to fail. Instead of reacting to breakdowns, teams can schedule repairs in advance to prevent production interruptions.
These systems are trained on historical data, then refined through continuous data collection. By recognizing subtle changes in vibration, temperature, or output speed, they provide alerts that allow teams to act precisely when needed.
Alongside monitoring, robotic process automation and AI-powered machines are improving how materials are handled and assembled.
Some factories deploy robots guided by deep learning algorithms, which are capable of identifying defects, adapting to object positions, and assisting workers with tasks that require speed and precision.
Some examples include:
- Quality control with vision-guided inspection tools
- Assembly line assistance using robotic arms
- Predicting inventory demand based on live production rates
- Load balancing in energy or material usage
The combination of artificial neural networks and predictive analytics allows for fast decisions with minimal human intervention, supporting safer environments and better use of skilled labor.
Transportation & Logistics: Reinforcement Learning & Computer Vision
In transportation and logistics, AI development is helping companies move goods more efficiently, plan routes with greater precision, and reduce dependency on manual tracking. AI is utilized in smart city applications to optimize resource management and improve urban services.
Two technologies are proving especially useful: reinforcement learning and computer vision.
Reinforcement learning allows AI systems to improve through trial and reward. These models simulate thousands of delivery scenarios, learning which routes, sequences, or methods produce the best outcomes in terms of time, fuel use, and delivery accuracy.
Over time, this process helps optimize decisions around fleet distribution, traffic conditions, and last-mile coordination, without requiring fixed rules.
For visual tasks, computer vision is applied to monitor vehicles, scan barcodes, verify loads, and assess road conditions in real time. Using deep learning models, these systems process video or image inputs to identify obstacles, detect anomalies, and confirm safe handoffs.
In warehouses, vision-based robotics assists with sorting, packaging, and inventory verification.
Common use cases include:
- Route optimization for delivery networks
- Cargo monitoring using real-time camera feeds
- Lane tracking and hazard detection in autonomous vehicles
- Robotic assistance in loading zones and fulfillment centers
These tools are most effective when trained on high-quality data and integrated with tracking systems. They also support resource management by reducing time lost to idle vehicles, delayed shipments, or human error in task execution.
Legal & Compliance: NLP & Document Intelligence AI
Legal and compliance teams are turning to AI development to manage increasingly large volumes of documentation, detect risks, and ensure regulatory accuracy without expanding headcount. AI tools have been used to enhance customer service through chatbots and virtual assistants that operate 24/7.
Two technologies leading this transformation are natural language processing (NLP) and document intelligence systems.
NLP-based models help companies extract meaning from legal language, summarizing contracts, flagging clauses, and surfacing inconsistencies. These systems interpret complex phrasing in human language, reducing time spent reviewing documents manually.
Document intelligence tools, powered by deep learning models, use structured and unstructured data to classify, tag, and organize files across formats. When integrated with case management software or cloud storage, they improve search accuracy, speed up audits, and help track legal obligations in real time.
Practical applications include:
- Contract analysis and clause comparison
- Regulatory document review across jurisdictions
- Discovery support during litigation
- Real-time policy monitoring in regulated industries
These solutions rely on well-trained machine learning algorithms, supported by high-quality data and updated to reflect the latest legal standards.
Education: Adaptive Learning AI & Generative AI
In education, AI development is unlocking new ways to personalize learning and improve how students engage with material. Through adaptive learning systems and generative AI, schools and edtech platforms are moving beyond static lesson plans and one-size-fits-all content.
Generative AI can create original content such as text, images, and videos in response to user prompts.
Adaptive learning tools adjust in real time based on how each student interacts with questions, videos, and activities.
Using machine learning models, these platforms track performance, identify learning gaps, and deliver material tailored to that student’s progress. The system learns from responses and guides the learner at the right pace, reducing frustration and reinforcing understanding.
On the content side, generative AI is helping educators create quizzes, practice questions, and summaries automatically.
Built on deep learning models and language-based AI algorithms, these tools understand curriculum structure and produce content aligned with specific goals. Some platforms use these models to simulate interactive tutoring experiences, offering explanations in different formats depending on the learner’s preferences.
Use cases include:
- Personalized study plans based on performance data
- AI-generated writing prompts or feedback suggestions
- Adaptive testing tools that adjust difficulty dynamically
- Virtual tutors who answer follow-up questions in plain language
Behind the scenes, these systems are powered by neural networks, training data, and ongoing data analytics. They do not replace instructors, but extend what educators can do, especially in large classrooms or remote learning environments.
Main Benefits of AI Systems
Faster Decision-Making with Real-Time Data
Modern AI systems process and respond to incoming data far faster than traditional workflows.
In environments where speed determines outcomes, finance, logistics, manufacturing, this advantage is hard to ignore. Through the use of machine learning algorithms and neural networks, these tools track, interpret, and react to high-volume inputs without pause.
Trained on quality data and connected to live feeds, AI models assist with forecasting, route planning, supply monitoring, and fraud detection.
In each case, the model’s ability to analyze data instantly helps decision-makers act without delay.
Examples include:
- Market trend reactions in financial platforms
- Real-time risk alerts in cybersecurity
- Adjustments in supply chains based on delivery status
Reduced Human Error in High-Stakes Environments
Mistakes in fields like aviation, healthcare, or infrastructure can lead to serious consequences. AI development is helping reduce these risks by introducing tools that follow consistent logic, maintain full attention on data, and avoid fatigue.
AI algorithms can embed ethical considerations and value choices into their programming, impacting decision-making. AI tools, when trained properly, minimize oversight by recognizing patterns and flagging inconsistencies early.
In healthcare, deep learning models identify anomalies in diagnostic scans. In transportation, automated systems support drivers with warnings, lane tracking, and braking assistance.
These systems don’t rely on memory or guesswork. Once built, they evaluate situations based on defined parameters, historical outcomes, and thousands of previous data points.
AI doesn’t eliminate all mistakes, but when applied correctly, it reduces the chance of oversight and improves safety in places where accuracy matters most.
Personalized Experiences at Scale
Delivering individual-level experiences used to require extensive manual effort.
Now, AI systems powered by machine learning algorithms can adjust responses, content, and recommendations for each user, without increasing the workload.
By analyzing behavior, past interactions, and unstructured data sets, these models create tailored outputs across industries. In e-commerce, they suggest products that match previous purchases.
In education, lessons are adjusted to the learner’s pace. In the media, they curate content based on user preferences and the time of day.
The engine behind this flexibility includes:
- Deep learning models capable of interpreting subtle usage patterns
- Text and voice understanding through natural language processing
- Real-time adjustments based on feedback or changes in context
Increased Efficiency in Routine Operations
Many tasks within business operations repeat daily, from responding to support tickets to generating reports. AI development helps reduce the burden of these functions by automating processes that don’t require human judgment.
Trained AI models process these tasks using clear logic and defined rules, making them ideal for structured decisions and data analysis.
This includes categorizing emails, handling basic requests, flagging anomalies, and sorting documents.
Tools used include:
- Workflow automation through API-driven bots
- Virtual assistants that respond to common questions
- Systems that fill forms or transfer data between platforms
How to Evaluate AI Fit for Your Industry
Does Your Business Generate High Volumes of Data?
Industries that collect large amounts of information, structured or unstructured, stand to gain the most from AI systems. Whether you're logging transactions, customer interactions, machine output, or logistics data, volume is a clear indicator of potential.
AI development becomes valuable when there is sufficient input to train models effectively. Machine learning algorithms rely on consistent access to high-quality data to identify patterns, test predictions, and produce reliable outcomes.
Examples include:
- Retail platforms tracking thousands of daily purchases
- Manufacturing teams recording sensor data from equipment
- Financial services processing transaction histories in real time
If your operation involves continuous data collection, there is likely an opportunity to use AI models for optimization, predicting, or anomaly detection. The more consistent the flow, the stronger the potential for automation and insight generation.
Are There Tasks That Require Repetition or Pattern Recognition?
Businesses often rely on employees to perform the same action hundreds of times each day, such as responding to similar messages, sorting entries, reviewing logs, or updating records.
These types of duties are well-suited for artificial intelligence.
AI systems excel at tasks that follow rules, repeat frequently, or involve identifying patterns in large datasets of information. Neural networks and deep learning models are especially effective at processing routine visual checks, language parsing, or form classification without fatigue or inconsistency.
Look for areas like:
- Processing invoices or receipts
- Analyzing support tickets for tone or urgency
- Reviewing sensor data for deviations in expected output
When tasks meet both criteria, high volume and recognizable patterns, it’s a clear indication that AI can reduce effort, speed up response times, and improve accuracy with minimal human intervention.
What Are the Risks of Manual Processing?
When tasks are performed by hand, especially in high-volume or time-sensitive environments, the potential for mistakes increases. Manual work often lacks consistency, and errors can lead to delays, compliance failures, or added costs.
AI systems offer a way to reduce those risks through logic-based, repeatable processes that maintain accuracy at scale.
Some of the most common pain points caused by manual workflows include:
- Inconsistent data entry across departments
- Delayed responses due to task bottlenecks
- Missed insights buried in overlooked reports
In contrast, machine learning models and AI algorithms perform the same task with the same standard each time. They don’t lose focus, skip steps, or mislabel information.
Do You Have Clear Metrics to Track AI ROI?
Successful AI development depends on more than the model itself, it also relies on knowing how to measure impact. Companies need clear, business-aligned benchmarks that reflect what the system is meant to improve.
Before starting any project, define what outcomes matter most.
That might be faster response times, fewer support requests, higher forecast accuracy, or increased output with the same team size. Once these targets are set, AI systems can be monitored through a combination of KPIs and real-time performance logs.
Strong signals of ROI readiness include:
- Clear baselines for current performance
- Available quality data for historical comparison
- A way to monitor AI output using analytics or logs
When goals are measurable, machine learning tools can be assessed properly and refined over time using insights from data analysis. Without metrics, it is difficult to determine whether automation is actually beneficial or merely adding complexity.
NerdHeadz Builds Industry-Specific AI Tools
At NerdHeadz, we help businesses bring AI development from idea to execution, focusing on real-world use cases instead of vague promises.
Our team works directly with companies across various industries, including logistics, education, retail, and services, to build tools that solve specific challenges, not just showcase the technology.
Each project begins by understanding your workflow.
We examine what is being done manually, where time is wasted, and which decisions rely on repetitive judgment. From there, we build AI systems tailored to those patterns, whether it means automating triage, generating content, analyzing incoming data, or delivering more responsive user experiences.
Some of the custom software we’ve built include:
- AI bots that qualify leads for real estate platforms
- Scheduling assistants for educators with built-in follow-up prompts
- Logistics dashboards with predictive insights and smart routing logic
- AI-powered document processors for compliance-heavy teams
- Onboarding flows with virtual assistants that adapt to user input
If your team needs industry-specific AI tools that go beyond experimentation, we’ll help you build something useful, measurable, and built for your next stage of growth, and to attract early adopters.
Conclusion
The industries seeing the most from AI development aren’t just adopting it because it’s trending, they’re using it to solve specific problems faster, more accurately, and with less manual effort. Whether it’s predicting machine failures, reducing customer wait times, or personalizing digital experiences, AI systems are helping teams improve outcomes and free up time for work that matters.
But success depends on building solutions that match your business, not a generic tool or one-size-fits-all model. That’s where we come in.
At NerdHeadz, we design industry-specific AI tools that deliver measurable impact from day one.
If you're ready to explore what AI can do for your team, book a call with us. We’ll help you find the right starting point, and build something that fits the way you already work.
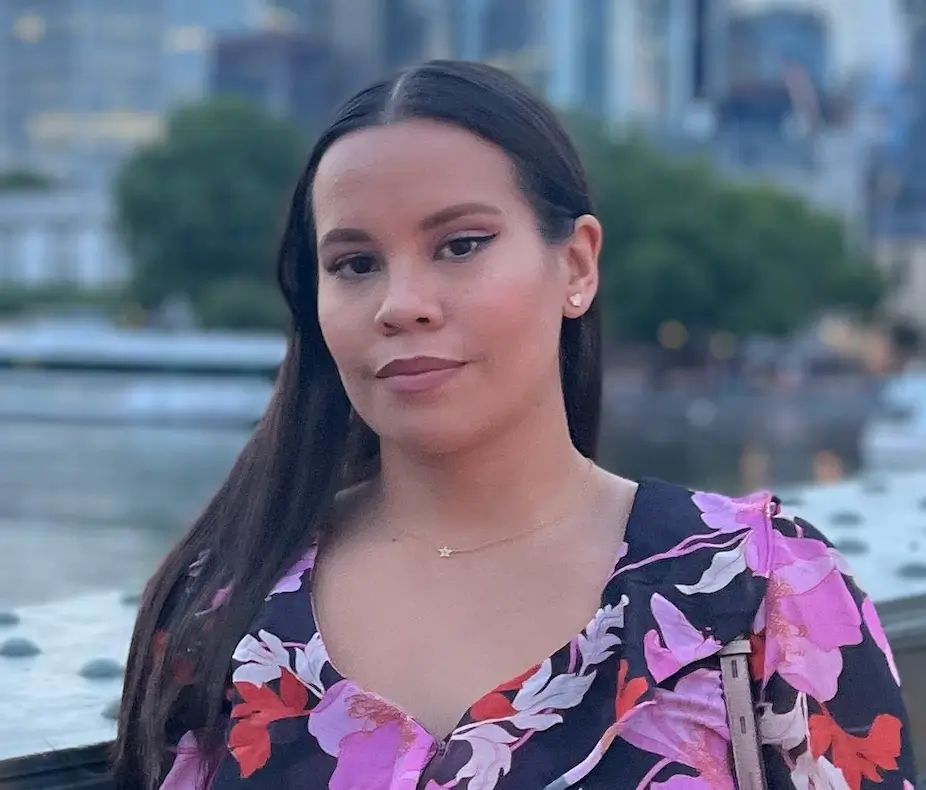
Luciani Zorrilla is a content marketer with experience in sales development, outbound sales, SEO, design, email marketing, and UX. She stands out in driving sustainable growth for tech startups through impactful SEO strategies and leading results-oriented marketing teams.